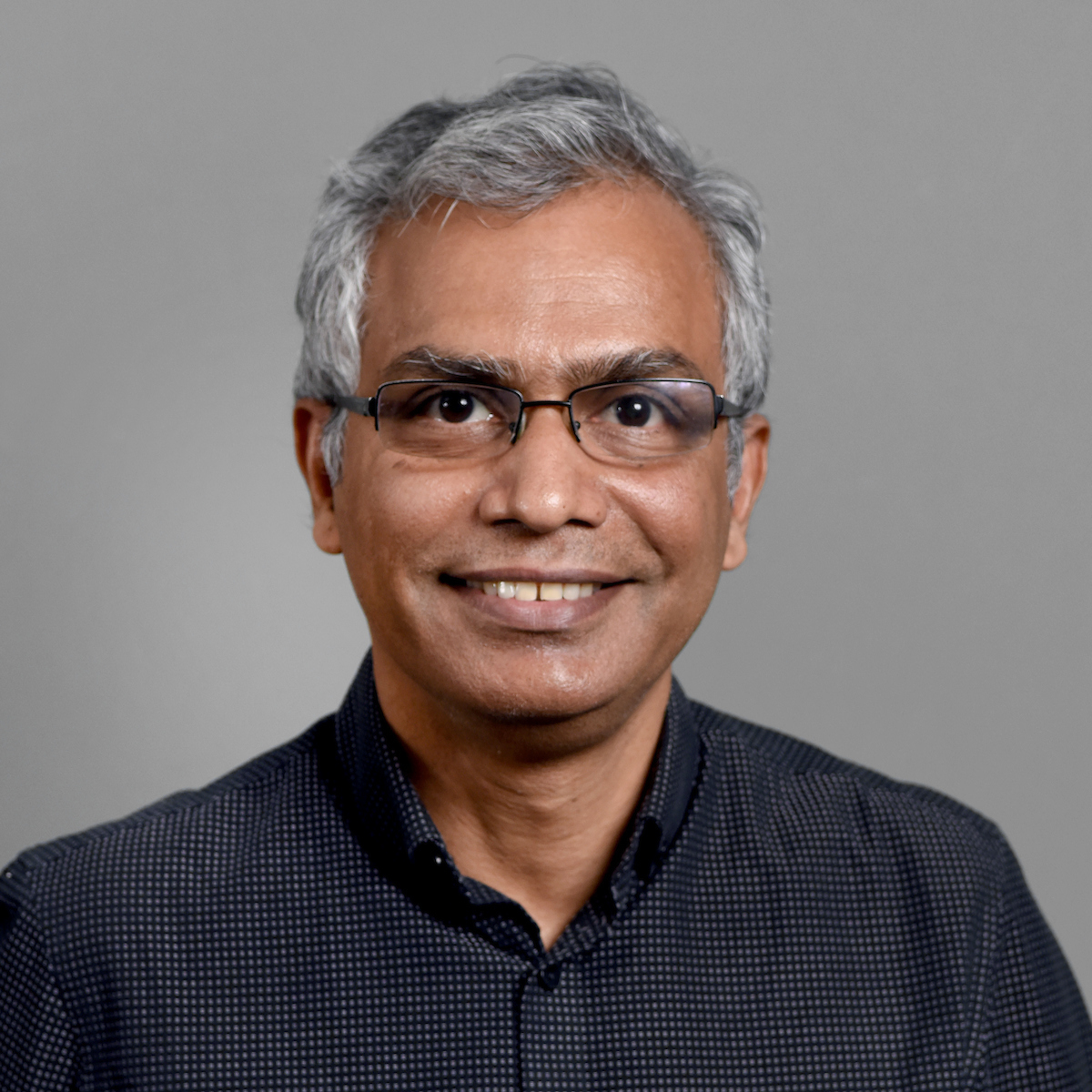
Subbarao Kambhampati
Subbarao Kambhampati (Rao) is a professor of Computer Science at Arizona State University. He received his B.Tech. in Electrical Engineering (Electronics) from Indian Institute of Technology, Madras (1983), and M.S.(1985) and Ph.D.(1989) in Computer Science (1985,1989) from University of Maryland, College Park. Kambhampati studies fundamental problems in planning and decision making, motivated in particular by the challenges of human-aware AI systems. Kambhampati is a fellow of AAAI and AAAS, and was an NSF Young Investigator. He received multiple teaching awards, including a university last lecture recognition. Kambhampati is the past president of AAAI and was a trustee of IJCAI. He was the program chair for IJCAI 2016 , ICAPS 2013, AAAI 2005 and AIPS 2000. He served on the board of directors of Partnership on AI. Kambhampati’s research as well as his views on the progress and societal impacts of AI have been featured in multiple national and international media outlets. URL rakaposhi.eas.asu.edu Twitter @rao2z
Synthesizing Explainable Behavior for Human-AI Collaboration
As AI technologies enter our everyday lives at an ever increasing pace, there is a greater need for AI systems to work synergistically with humans. This requires AI systems to exhibit behavior that is explainable to humans. Synthesizing such behavior requires AI systems to reason not only with their own models of the task at hand, but also about the mental models of the human collaborators. Using several case-studies from our ongoing research, I will discuss how such multi-model planning forms the basis for explainable behavior.
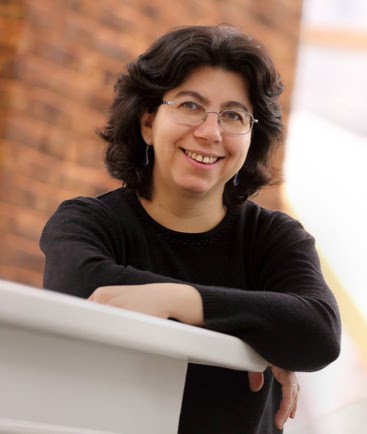
Doina Precup
Doina Precup splits her time between McGill University/Mila, where she holds a Canada-CIFAR AI chair, and DeepMind, where she leads the Montreal research team formed in 2017. Her research focuses on reinforcement learning, deep learning, time series analysis, and diverse applications of machine learning with a special focus on health care. She completed her BSc/Eng (1994) degree in computer science at the Technical University Cluj-Napoca, Romania, and her MSc (1997) and PhD (2000) degrees at the University of Massachusetts, Amherst, where she was a Fulbright scholar. She became a senior member of AAAI in 2015, a Canada Research Chair in 2016 and a Senior Fellow of CIFAR in 2017. Doina Precup is also involved in organizing activities aimed at increasing diversity in machine learning, such as the AI4Good summer lab and the Eastern European Machine Learning school.
Building Knowledge For AI Agents With Reinforcement Learning
Reinforcement learning allows autonomous agents to learn how to act in a stochastic, unknown environment, with which they can interact. Deep reinforcement learning, in particular, has achieved great success in well-defined application domains, such as Go or chess, in which an agent has to learn how to act and there is a clear success criterion. In this talk, I will focus on the potential role of reinforcement learning as a tool for building knowledge representations in AI agents whose goal is to perform continual learning. I will examine a key concept in reinforcement learning, the value function, and discuss its generalization to support various forms of predictive knowledge. I will also discuss the role of temporally extended actions, and their associated predictive models, in learning procedural knowledge. Finally, I will discuss the challenge of how to evaluate reinforcement learning agents whose goal is not just to control their environment, but also to build knowledge about their world.
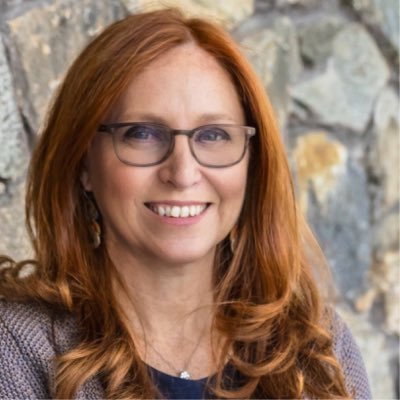
Francesca Rossi
Francesca Rossi is the IBM AI Ethics Global Leader, a distinguished research scientist at the IBM T.J. Watson Research Centre, and a professor of computer science at the University of Padova, Italy.
Francesca’s research interest focuses on artificial intelligence, specifically constraint reasoning, preferences, multi-agent systems, computational social choice, and collective decision making. She is also interested in ethical issues surrounding the development and behavior of AI systems, in particular for decision support systems for group decision making. A prolific author, Francesca has published over 190 scientific articles in both journals and conference proceedings as well as co-authoring A Short Introduction to Preferences: Between AI and Social Choice. She has edited 17 volumes, including conference proceedings, collections of contributions, special issues of journals, and The Handbook of Constraint Programming.
Francesca is both a fellow of the European Association for Artificial Intelligence (EurAI fellow) and also a 2015 fellow of the Radcliffe Institute for Advanced Study at Harvard University. A prominent figure in the Association for the Advancement of Artificial Intelligence (AAAI), at which she is a fellow, she has formerly served as an executive councilor of AAAI and currently co-chairs the association’s committee on AI and ethics. Francesca is an active voice in the AI community, serving as Associate Editor in Chief of the Journal of Artificial Intelligence Research (JAIR) and as a member of the editorial boards of Constraints, Artificial Intelligence, Annals of Mathematics and Artificial Intelligence (AMAI), and Knowledge and Information Systems (KAIS). She is also a member of the scientific advisory board of the Future of Life Institute, sits on the executive committee of the Institute of Electrical and Electronics Engineers (IEEE)’s global initiative on ethical considerations on the development of autonomous and intelligent systems, and belongs to the World Economic Forum Council on AI and robotics.
Preferences and Ethical Priorities: Thinking Fast and Slow in AI
In AI, the ability to model and reason with preferences allows for more personalized services. Ethical priorities are also essential, if we want AI systems to make decisions that are ethically acceptable. Both data-driven and symbolic methods can be used to model preferences and ethical priorities, and to combine them in the same system, as two agents that need to cooperate. We describe two approaches to design AI systems that can reason with both preferences and ethical priorities. We then generalize this setting to follow Kahneman's theory of thinking fast and slow in the human's mind. According to this theory, we make decision by employing and combining two very different systems: one accounts for intuition and immediate but imprecise actions, while the other one models correct and complex logical reasoning. We discuss how such two systems could possibly be exploited and adapted to design machines that allow for both data-driven and logical reasoning, and exhibit degrees of personalized and ethically acceptable behavior.
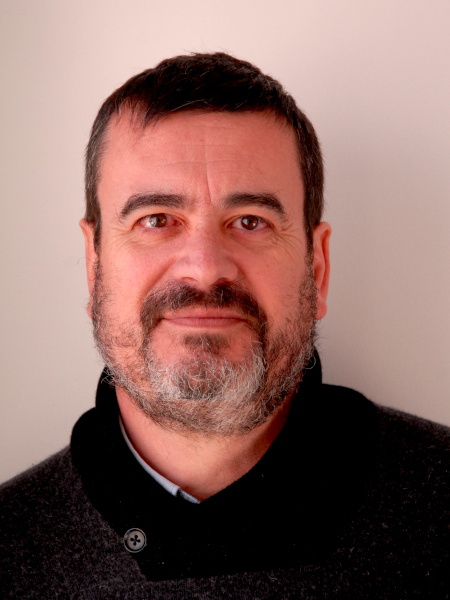
Carles Sierra
Carles Sierra is a Research Professor of the Artificial Intelligence Research Institute (IIIA-CSIC) in the area of Barcelona. He is currently the Vice-Director of the Institute. He received his PhD in Computer Science from the Technical University of Barcelona (UPC) in 1989. He has been doing research on Artificial Intelligence topics since then. He has been visiting researcher at Queen Mary and Westfield College in London (1996-1997) and at the University of Technology in Sydney for extended periods between 2004 and 2012. He is also an Adjunct Professor of the Western Sydney University. He has taught postgraduate courses on different Ai topics at several Universities: Université Paris Descartes, University of Technology, Sydney, Universitat Politècnica de València, and Universitat Autònoma de Barcelona among others.
He has contributed to agent research in the areas of negotiation, argumentation-based negotiation, computational trust and reputation, team formation, and electronic institutions. These contributions have materialised in more than 300 scientific publications. His current focus of work gravitates around the use of AI techniques for Education and on social applications of AI. Also, he has served the research community of MAS as General Chair of the AAMAS conference in 2009, Program Chair in 2004, and as Editor in Chief of the Journal of Autonomous Agents and Multiagent Systems (2014-2019). Also, he served the broader AI community as local chair of IJCAI 2011 in Barcelona and as Program Chair of IJCAI 2017 in Melbourne. He has been in the editorial board of nine journals. He has served as evaluator of numerous calls and reviewer of many projects of the EU research programs. He is an EurAI Fellow and was the President of the Catalan Association of AI between 1998-2002.
Responsible Autonomy
The main challenge that artificial intelligence research is facing nowadays is how to guarantee the development of responsible technology. And, in particular, how to guarantee that autonomy is responsible. The social fears on the actions taken by AI can only be appeased by providing ethical certification and transparency of systems.1 However, this is certainly not an easy task. As we very well know in the multiagent systems field, the prediction accuracy of system outcomes has limits as multiagent systems are actually examples of complex systems. And AI will be social, there will be thousands of AI systems interacting among themselves and with a multitude of humans; AI will necessarily be multiagent.
Although we cannot provide complete guarantees on outcomes, we must be able to define with accuracy what autonomous behaviour is acceptable (ethical), to provide repair methods for anomalous behaviour and to explain the rationale of AI decisions. Ideally, we should be able to guarantee responsible behaviour of individual AI systems by construction.
I understand by an ethical AI system one that is capable of deciding what are the most convenient norms, abide by them and make them evolve and adapt. The area of multiagent systems has developed a number of theoretical and practical tools that properly combined can provide a path to develop such systems, that is, provide means to build ethical-by-construction systems: agreement technologies to decide on acceptable ethical behaviour, normative frameworks to represent and reason on ethics, and electronic institutions to operationalise ethical interactions. Along my career, I have contributed with tools on these three areas [1, 2, 5]. In this keynote, I will describe a methodology to support their combination that incorporates some new ideas from law [3], and organisational theory [4].